Colloquium: The mechanistic analysis of founder virus data in challenge models
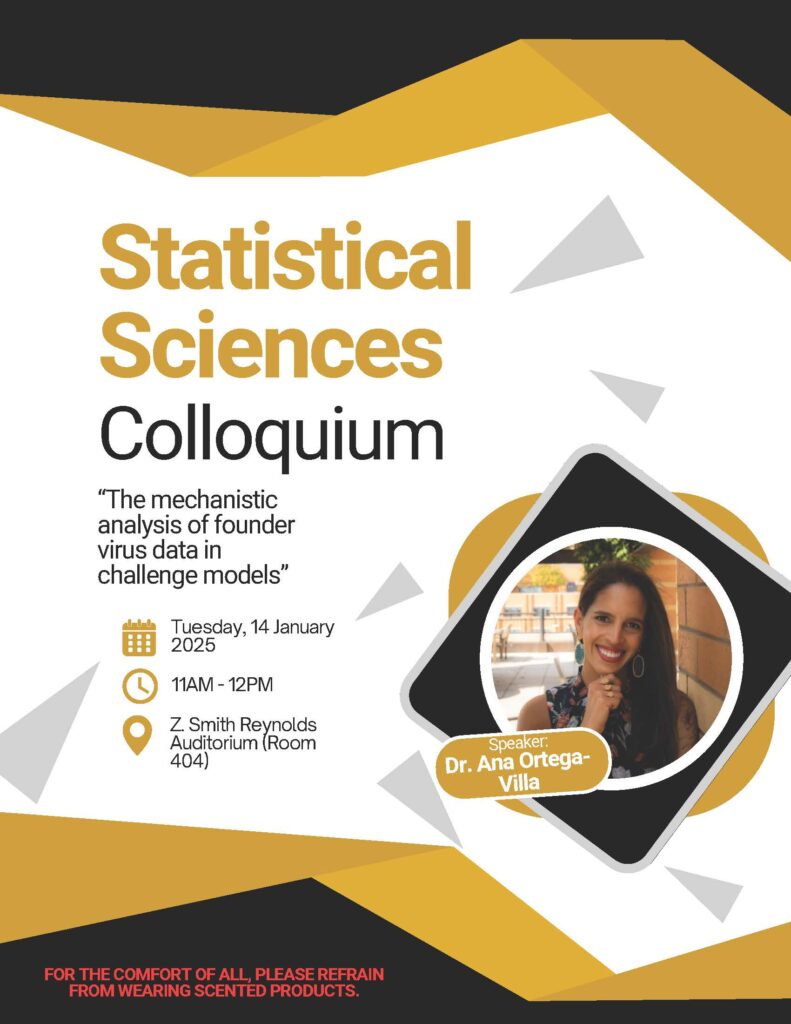
Dr. Ana Maria Ortega-Villa will be presenting at 11 AM in the Z. Smith Reynolds (ZSR) Auditorium, Room 404. Dr. Ana M. Ortega-Villa joined the Biostatistics Research Branch (BRB) in 2018 and serves as a mathematical statistician. Prior to joining the BRB, Dr. Ortega-Villa obtained her Ph.D. in Statistics from Virginia Tech and completed post-doctoral fellowships at both the Eunice Kennedy Shriver National Institute of Child Health and Human Development and the National Cancer Institute. Her interests include longitudinal data, mixed models, vaccines, immunology, research capacity building in developing countries, statistics education, and diversity and inclusion initiatives.
Dr. Ortega-Villa’s talk is entitled “The mechanistic analysis of founder virus data in challenge models.” An abstract is as follows.
Abstract: Repeated low-dose challenge studies provide valuable information when evaluating candidate vaccines since they resemble the typical exposure of natural transmission and inform on the number of exposures prior to infection. Traditionally, the number of challenges to infection has been used as the outcome. This work uses the number of infecting viruses, or founder viruses at the time of infection, to more efficiently characterize a vaccine’s mechanism of action. The vaccine mechanisms of action we consider are a Null mechanism (the vaccine offers no protection), a Leaky mechanism in which the number of founder viruses is reduced by some factor in vaccinated subjects, the All-or-None mechanism in which the vaccine randomly provides either complete protection or no protection in vaccinated subjects, and a Combination mechanism with both Leaky and All-or-None components. We consider two discrete marked survival models where the number of founder viruses follows a Poisson distribution with either a fixed mean parameter (Poisson model), or a random mean parameter that follows a Gamma distribution (negative binomial model). We estimate the models using maximum likelihood and derive likelihood ratio testing procedures that are accurate for small samples with boundary parameters. We illustrate the performance of these methodologies with a data example of simian immunodeficiency virus on nonhuman primates and a simulation study.