Colloquium: Bayesian nonparametric regression models for insurance claims data
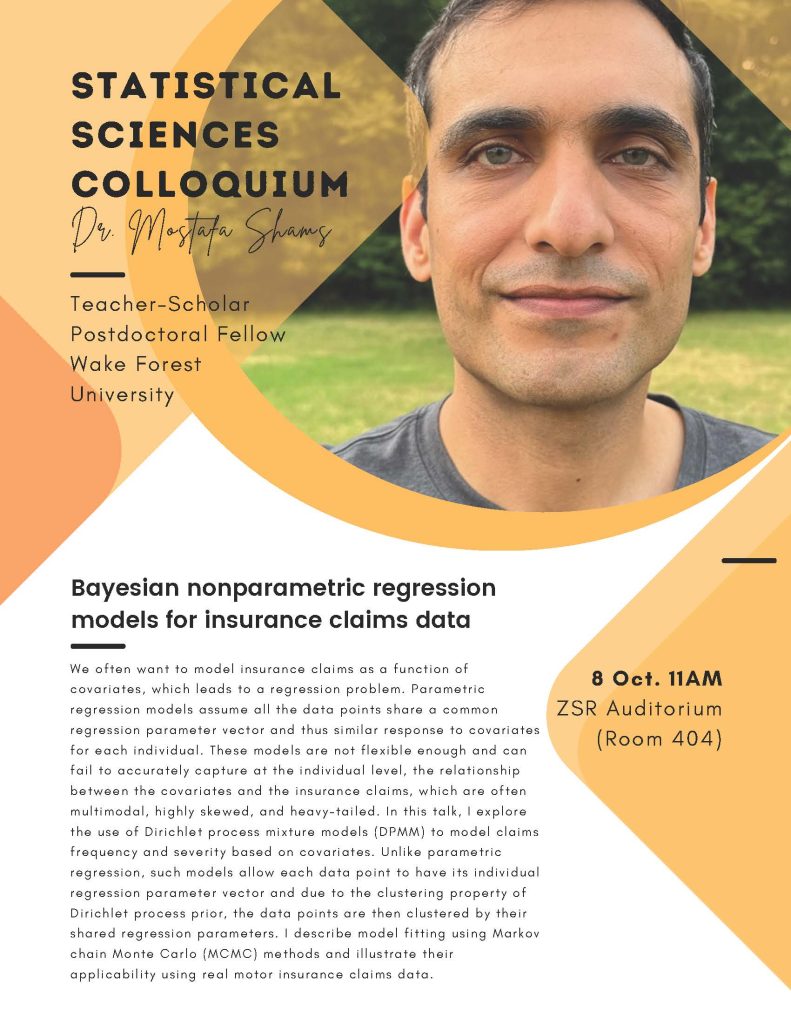
Statistical Sciences Colloquium
Dr. Mostafa Shams Teacher-Scholar Postdoctoral Fellow
Wake Forest University
Bayesian nonparametric regression models for insurance claims data
8 Oct. 11AM, ZSR Auditorium (Room 404)
We often want to model insurance claims as a function of covariates, which leads to a regression problem. Parametric regression models assume all the data points share a common regression parameter vector and thus similar response to covariates for each individual. These models are not flexible enough and can fail to accurately capture at the individual level, the relationship between the covariates and the insurance claims, which are often multimodal, highly skewed, and heavy- tailed. In this talk, I explore the use of Dirichlet process mixture models (DPMM) to model claims frequency and severity based on covariates. Unlike parametric regression, such models allow each data point to have its individual regression parameter vector and due to the clustering property of Dirichlet process prior, the data points are then clustered by their shared regression parameters. I describe model fitting using Markov chain Monte Carlo (MCMC) methods and illustrate their applicability using real motor insurance claims data.