Colloquium: Optimal Dynamic Treatment Rule Estimation and Evaluation with Application to Criminal Justice Interventions in the United States
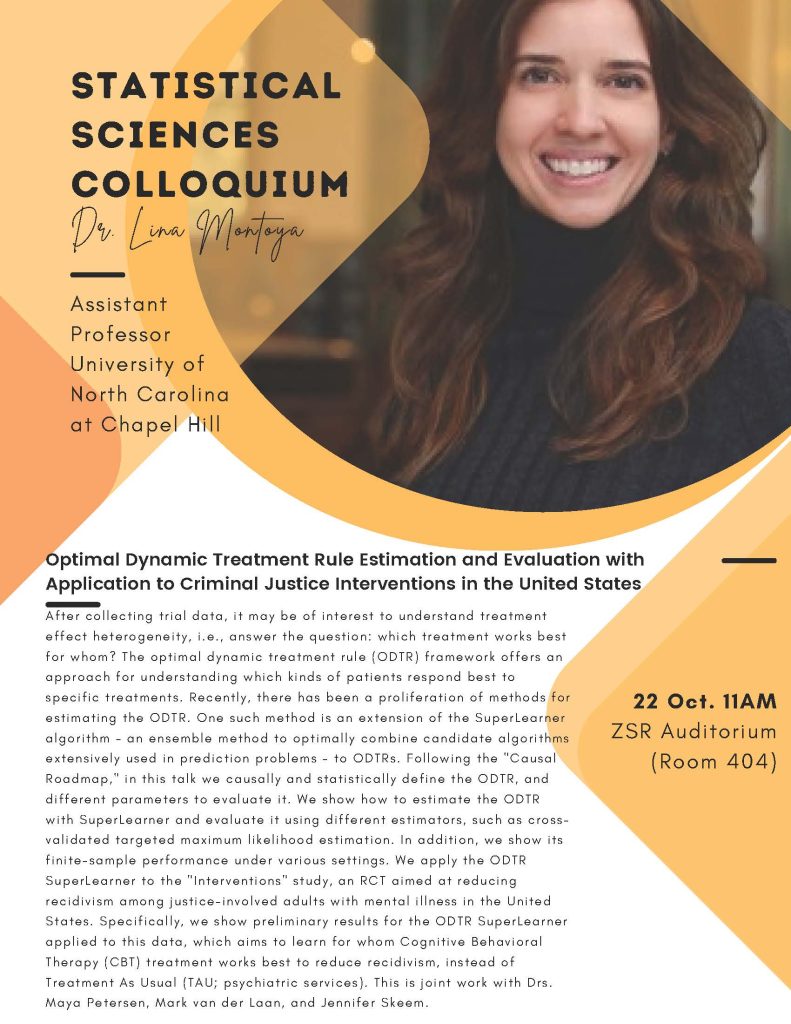
Dr. Lina Montoya, Assistant Professor
University of North Carolina at Chapel Hill
22 Oct. 11AM, ZSR Auditorium (Room 404)
After collecting trial data, i t may be of interest to understand treatment
effect heterogeneity, i.e., answer the question: which treatment works best for whom? The optimal dynamic treatment rule (ODTR) framework offers an approach for understanding which kinds of patients respond best to specific treatments. Recently, there has been a proliferation of methods for estimating the ODTR. One such method i s an extension of the Super Learner algorithm – an ensemble method to optimally combine candidate algorithms extensively used in prediction problems – to ODTRs. Following the ” Causal Roadmap,” in this talk we causally and statistically define the ODTR, and different parameters to evaluate it. We show how to estimate the ODTR with Super Learner and evaluate it using different estimators, such as cross- validated targeted maximum likelihood estimation. In addition, we show its finite- sample performance under various settings. We apply the ODTR Super Learner to the ” Interventions” study, an RCT aimed at reducing recidivism among justice- involved adults with mental illness in the United States. Specifically, we show preliminary results for the ODTR Super Learner applied to this data, which aims to learn for whom Cognitive Behavioral Therapy ( CBT) treatment works best to reduce recidivism, instead of Treatment As Usual ( TAU; psychiatric services). This i s joint work with Drs. Maya Petersen, Mark van der Laan, and Jennifer Skeem.